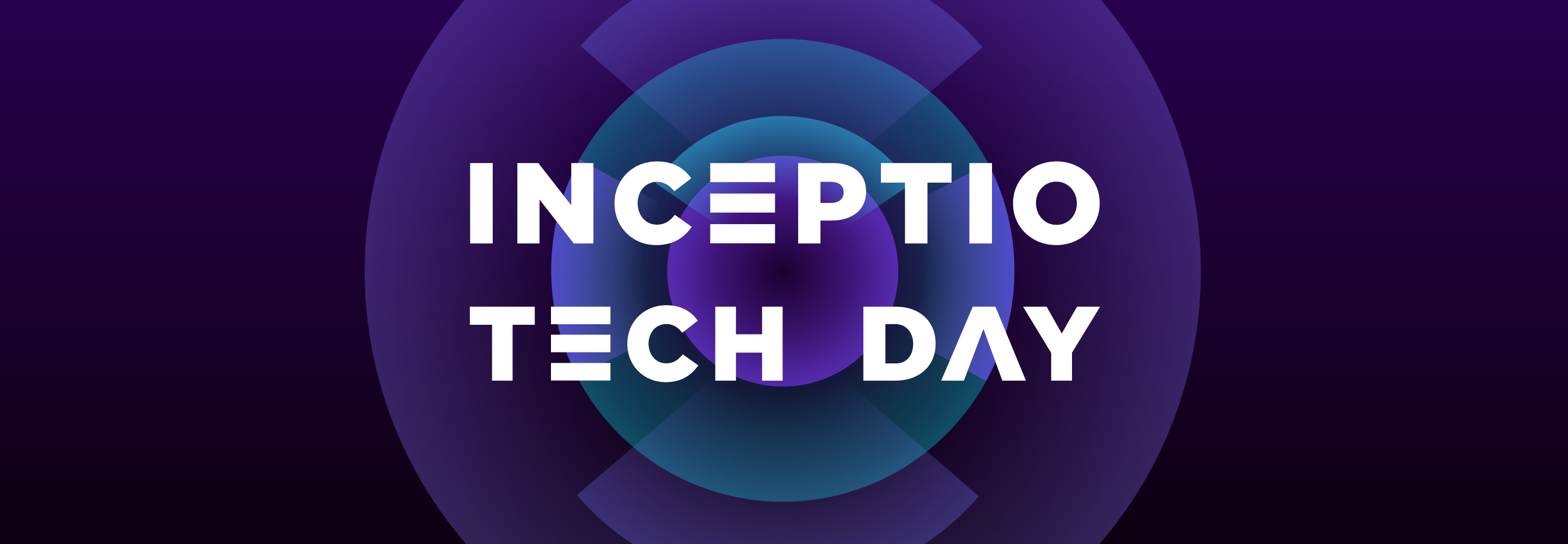
Inceptio Tech Day: The technical road map from mass production to driverless
2022-09-01
On September 1, 2022, Inceptio held the first Tech Day and unveiled the three-stage technical road map from mass production to driverless. It also deeply shared the core technologies and develop process behind the three-year mass production journey, and released the "White Paper on Mass Production of Autonomous Trucks".
Julian Ma, founder and CEO of Inceptio Technology, said, "The autonomous driving industry has entered a new stage. The technical focus has shifted from algorithm exploration to mass production of pre-loaded autonomous driving system. Now we see a shared view that the technology, system, and experience of autonomous vehicles’ mass production have become scarce capabilities in the industry. Inceptio has adhered to the technology strategy of full-stack propriety, mass production orientation, and industrial integration since its establishment. After nearly three years of joint development by Inceptio and top truck OEMs in China, the heavy-duty truck pre-loaded with Inceptio Xuanyuan autonomous driving system has been successfully mass produced by the end of 2021. With strong market demand of autonomous trucks, the commercial operation mileage of autonomous truck has rapidly exceeded 6 million kilometers. Through this tech day, we share mass production practices with the whole industry, and look forward to a consensus of system building, technology exploration and industry progress. "
3-Stage technical road map from mass production to driverless
With the mass production of Inceptio Xuanyuan system 1.0, Inceptio's full-stack proprietary technology has entered the 2.0 stage, with breakthroughs in core technologies. In terms of algorithm evolution, Inceptio has achieved three breakthroughs: the pre-fusion perception framework applying multi-mode and multi-view transformer, the tightly coupled planning and control integration architecture, and the multi-time-scale fuel-saving system. As for hardware iteration, our self-developed gen-ii automotive-grade computing platform has lower cost and continues to lead in performance. Regarding data assets, the innovative data closed loop with enhanced shadow mode is used to strengthen the technological leadership.
At the perception level, Inceptio adopts the pre-fusion perception framework applying the multi-mode multi-view transformer. It can switch from the perspective view to the BEV view, and fully fuse the feature maps of different data sources, and then use the long short-term memory (LSTM) time series fusion network to obtain the perception result. Thus, it would effectively deal with the unique challenges of heavy trucks in line-haul logistics, such as sensing small objects at a distance, special-shaped vehicles, etc. At the same time, it helps reduce calculation amount by 50% with the lightweight model, and the NPU is used to accelerate the traditional CV algorithm (for preprocessing), which is 100 times faster.
The difficulty of planning and control is to meet the five major commercial operation requirements - safety, accuracy, comfort, durability, and economy at the same time. This inevitably brings about three major contradictions between precise modeling and multi-vehicle adaptation, precise control and durable economy, planning controls stratification and fusion. Inceptio has creatively developed a PNC architecture that integrates planning and control, realizing the tight coupling of forecasting, decision-making, planning and control, and eventually helps finding the optimal solution for demanding operational requirements.
In terms of fuel-saving algorithms, Inceptio has comprehensively optimized fuel consumption at sub-second, second, minute, and hour levels. In particular, the hour-level breakthroughs have been made in the vehicle-cloud synergy global velocity planning algorithm. A GNN (Graph Neural Network) with key waypoints as nodes is established in the cloud, with historical experience data and real-time traffic data being used to achieve optimal speed recommendation. In actual operation, the average fuel saving is 2-5% compared with top skilled drivers, approaching the upper limit of 7% fuel saving.
At the core hardware level, Inceptio's proprietary computing platform has entered the gen-ii stage, with smaller size, better performance, and lower cost. The computing power of the single board is as high as 256 TOPS, and the scalable architecture supports expansion to more than 1000 TOPS. The platform is based on the TSN protocol and can achieve a time synchronization accuracy of 30ns, which is industry leading.
Along with the large-scale production and accelerated operational deployment, the mileage of autonomous trucks in commercial operation has increased rapidly. Inceptio has established the industry's first closed-loop data system for autonomous trucks, turning data assets into technological advantages. Inceptio's innovative enhanced shadow mode, compared to the traditional shadow mode, provides the ability to collect and evaluate long-term behaviors (such as fuel consumption-related behaviors, etc.) required for L4 iteration. It helps realize efficient algorithm iteration of real-time A/B Test, and reduce data collection costs while improving data validity and accuracy.
With these breakthroughs, an overall leading and highly scalable Inceptio Xuanyuan Autonomous Driving System 2.0 has been on its way towards mass production.
For driverless autonomous driving technology, with the belief that the current autonomous driving technology architecture is likely to run into bottlenecks, Inceptioproposes a new concept of autonomous driving architecture in Inceptio Xuanyuan System 3.0. It can remove the artificially designed boundaries between the sub-modules of the autonomous driving system and replace it with an end-to-end deep neural network. Combined the technology advantages of Learning (DRL) and Neural Radiation Field (NeRF), the NeDFS (Neural Driving Field Simulator) Ultimate Autonomous Driving Simulator, will be used for training end-to-end models, and will realize self-evolution of autonomous driving capabilities, and eventually become unmanned.